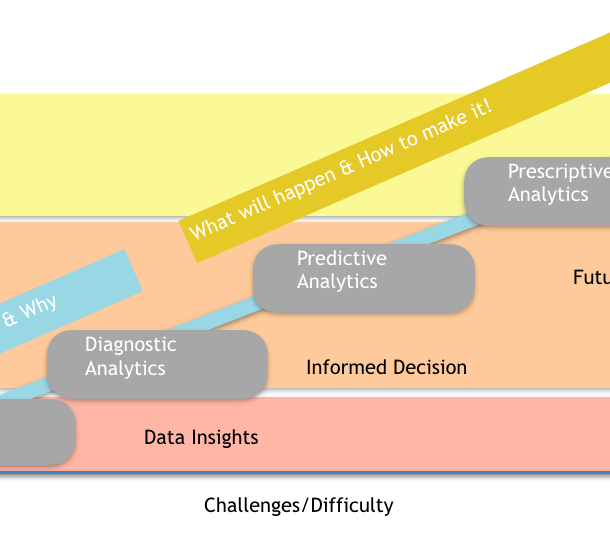
- +41 41 201 88 44
- [email protected]
- Mon-Fri 8am - 6pm
When people hear AI, they often think about automated machines, robots and magical devices. In reality, AI is much more invisible, wide spread, valuable and mundane tool and yet simple in implementation for business. Another misconception is that high profile research projects can be applied directly to any business situation. AI applied to the right business use case and executed in a right way can create high return on investments (ROIs): for instance through automation or precise prediction of customer segmentation or churn rates or future demand for a product. But it does take more effort in terms of thought, resources and right execution.
The drive for digitalisation and automation are the main forces for pushing forward into cognitive approaches to enhance the human experience further. Though there has been much efforts towards cognitive insights and data based knowledge and discovery, humans are at times overwhelmed with how to handle complex relations in the information and derive valuable insights. In addition to this the ability to comprehend, complex intelligent decisions in knowledge intense businesses.
The key functions of HR like to scout for talent and hiring them can be a challenge for big enterprises. And after hiring the next steps would be to engage the new hire and retain the talent till the investment pays off. And also from the individuals perspective how to grow and serve the organisation.
In which aspects of these functions can the AI be of help and how? Matching of the candidates using natural language processing based algorithms with AI is better than the traditional key word based searches. Thus sharing the skill matching for the roles across the organisation can be communicated to the candidate to provide a transparent and positive recommendations based on analysis.
Further, deploying chatbots to give candidates a better experience with respect to the organisation’s needs and its behaviour. Even smarter compensation planning can be implemented based on the set targets and also by individualising the recommendations for personalised learning. Finally, it is possible to have a 24X7 HR availability employee interaction in cases of emergency and need.
Recently many organisations realised the importance of CRM with AI enabled as a transformation engine to increase the performance of the sales teams. This is now more a norm than an exception for many industries to introduce some form of AI into the workflow to improve the outcomes. First steps being retrieving data on demand and data integration for the sales team is the most time limiting step. It also adds to the barrier for a wider adoption of CRM systems. But once the data is in place it makes more value. An important aspect of data collection is quality and integrity of the data. Pre-existing data for many of the corporations is either incomplete with irregularities, duplications etc. Therefore the current CRM’s are trying to implement automation as a first step before deriving intelligence from the available data. Therefore transcribing customer interaction in an automated way using natural language processing (NLP) transcription will enhance the capabilities of CRM systems.
Secondly, the modern sales force in an organisation interacts in a very different way with the customers than earlier times. Most of the interactions with the customers are online and virtually all are voice mediated. In such cases an inline on call voice analysis for sentiment and emotional state of the customer will help build trust and value to the sales pitch. Having all the data in a database is of high value to the AI system to derive valuable leads by giving predictive scores to each lead. Thus built knowledge base will be a valuable resource along with the other customer data to build customer specific recommendations for the sales team.
Logistics and supply chain management generates a vast amount of data, mainly generated by monitoring and quality controlling of vast resources and flow raw materials and goods. Traditionally most of the supply chain management had lots of data that is exiting in every enterprise. Though the data was structured and available for analytics, the real use cases to generate business value was difficult. Recently, that has changed with the advent of AI and machine learning algorithms, there has been intelligence in a real value process that gain from the application of AI, like predictive demand forecasting and operational process optimisation. The former is widely adapted across the logistics and supply chains where as the later is evolving into an essential element for improving operations and processes using sensor technology like Internet of Things(IoT). Logistic gains for implementing intelligence and ML in their day to day operations has shown very high ROI.
For many companies the realtime analytics also play the pivotal role in making decisions on the go. Abundance of reliable and cheap sensor technologies have generated vast amounts of data using IoT and not every organisation is equipped with the necessary know how and the infrastructure to derive value. Data generated in this way holds considerable potential for optimisation. A central challenge is the intelligent use of data using both Big Data and IoT technologies and building applications levying realtime analytics and providing insights for making right decisions. A first step towards this is to test and perform evaluations to select the right architecture and enable end to end solution framework.
Operational optimisation using AI is a challenging venture that needs good understanding of the business processes in an organisation and a clear data representing the current timelines and the capacity of the company. Having dynamic capacity challenges adds on to the uncertainty. In such scenarios building a safe capacity in line with the projected work orders or demand is a plausible solution. Reduction in operational cost and throughput time are data driven and can be modelled with more accurate understanding of business process and discover deviations and non-compliance.
Cashflow prediction is one of the essential financial elements that plays key role in driving a companies health and future growth. With the availability of technology daily, week or monthly forecasts have become easy to implement and have an effect on day to day operations. Providing cash flow forecasts under critical circumstances for the management is the key in making decisive actions. It is important to know if they are able to make payroll next month? Does the owner of the business wants to evaluate the possibility or affording to take on new project based on the current working capital requirements and expenditures. And how, based on the current growth and yearly forecasts, can increase dividend payouts or not. It is well known that poor cash flow and decisions made based on this can kill large and small businesses alike. What’s critical is that a forecast offers possibilities, typically a best-case, worst-case, and probable scenario set to take the next steps.
Of course, it is hard to implement non-predictable sales outcomes, expenditures, or miscellaneous events that occur from time to time. And therefore need to be taken into consideration for a forecast to be meaningful in the real world. With this in mind, modern forecasting algorithms come with a lot more flexibility to simulate seasonality, holidays, unexpected and tragic events. There are features built in allowing for manual adjustments that address special situations if necessary along with forecast errors. Collectively these contribute to information asymmetry and can be taken into consideration for modelling the data in improving future forecasts. Rolling forecasts adapting to the changing economic scenarios are the challenging forecasting tasks. Especially, in the current scenario it is difficult to model the cash flow using classical sensitivity analysis and what if scenarios. Implementing AI is not only to predict future growth or forecast cashflows, but it can be a valuable instrument in deciphering the key variables that are affecting a specific product or process in place which is either the ‘catalyst’ in building business or a potential ‘fatalyst.’ (fatal for business). And there are a large number of applications in financial and banking institutions, which require lot more understanding of banking processes and specialised knowledge of the banking industry.
In reality AI is going to be omnipresent in every device of future. And every application on many devices will also be using AI in oneway or the other. Therefore the growth potential in implementing AI enterprise wise is going to get quick ROI. To Understanding and identifying the key business processes that will be profited by the implementation of AI, the use of experts is a good first step to come to a point of aggregating possibilities how can they be envisioned. It is important to keep in mind that data is the key. Its quality, completeness and reliability are paramount to build valuable AI applications in the organisation. If non- existent then implementing quality data acquisition is a measure to be prepared for the future growth.
The key to this is to decide how to chose the data science integration or make it widely accepted in the organisation. And finally, primary job is to promote the culture of data awareness across the enterprise. One question that stands out is the dilemma to use available AI software solutions vs building your own data science and machine learning teams. This needs a thorough evaluation of the possible use cases and the value the application of AI will bring in. And more importantly the resources and the know how in-house to get into such a venture.
Once you achieve the organisational preparedness and acceptance to data driven decisions, the final vision is to achieve intuitive flow of data across the enterprise from different databases and departments to the front end of users and decision makers. Finding the collaborative forces who have a know how to evaluate the use case and understand by interacting with the business people and collaboratively building the AI together is a challenge. Though the business processes for each enterprise are different, their overall data architectures and information flow patterns have similarities. Therefore leveraging the expertise in the AI and business from external experts or consultants will give first glimpse into the possibilities.
Expertise in the business and technology together are difficult to find. Thats why it is important to initiate a small proof of concept study after surveying the possible use cases in consultation with the business specialists and AI experts and consultants. Then collaboratively do a quick development of a minimum valuable product (MVP) which is viable. First step is the evaluation of the use case, the available resources and the data availability. Second, bring in experts who are both business and technologically savvy. And decide on a simple project among the selected use cases which can be completed in few weeks to 3 months. Third, decide and set the key performance indicators (KPI) in consultation with both the business people, stake holders and AI experts, who are of paramount importance for the success of the MVP. Once set out will all the required parameters evaluate at every check point set over 2 to 3 weeks to refine the KPI’s to reflect upon the real world progress and as a measure of success. Finally, do a careful evaluation of the success from both the business side as well as the technical side.
It is essential to have an inclusive approach for designing the initial use cases with the business outcome in mind. Using the existing products see how AI can be quickly implemented by utilising existing infrastructure and validate the product. MVP is the down payment on a larger vision. It is done as you dont have the time to fix in every nut and bolt of the process of implementing AI pipelines across all products. So pick a product that is moving and see if you can give it a tool to increase the pace or enhance functionality. It could be an internally developed AI software or a third party vendor software tools. After successfully finishing the first MVP, you have a good head start into the enterprise AI and ready for future.
Steinentorstrasse 35 , 4051 Basel, Switzerland