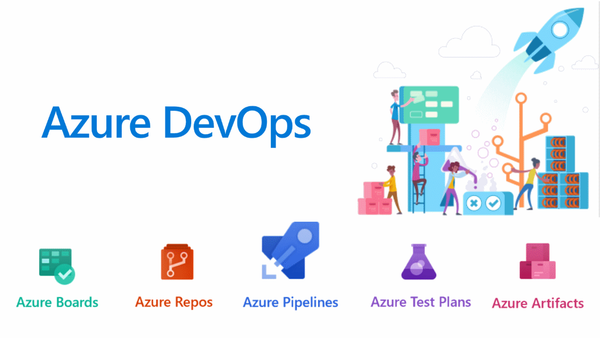
- +41 41 201 88 44
- [email protected]
- Mon-Fri 8am - 6pm
Artificial Intelligence(AI) or Machine intelligence is a computational process by machines towards learning and understanding the environment and perform actions that maximize its chances of success to achieve a goal using pieces of code. Traditionally it stems from the combination of statistics and computing power to learn and perform things not doable with traditional computers.
Some of the real world examples of AI include driverless cars, speech recognition and real time language translation, simulating strategic games, designing new drugs and reinforcing learning behaviour in robots etc. Using an algorithm involving above mentioned criteria of AI predict the behaviour or future outcomes of a business process like revenue, sales or customer churn rates, language translation etc.
The reason for the implementation of AI in an organisation is multifold. Analytics give insights to the people and the management about the current state of affairs of the business. Primarily the type of analytics applied are broadly divided into Descriptive analytics, diagnostic analytics, predictive analytics and prescriptive analytics. These various levels of statistical applications give valuable insights to the management in driving towards a sustainable revenue and future growth.
Descriptive analytics provide the insights into the statical aspects of data that define how the historical data is distributed and what can be prepared for further data analysis. Descriptive analytics based on the data give companies overview of what has happened in the business over the period of time. This preliminary analysis is the primary data to understand comprehensively and accurately categorising features like total income versus the available products or different regions, total stock available, sales momentum etc.
This is the next level of drill down analysis after the descriptive analysis. Detailed root cause analysis of the situation fall under this category. Mostly Data analysts or BI professionals handle this kind of analysis and drill down for understanding the root cause and analyse outcomes. Give an in-depth analytics and categorisation of the results.
Predictive analytics is the next evolutionary step in analytics, where we have the power to predict future and be prepared for the possible outcomes. And this relies mostly on the historical data and the quality of the data the enterprise has and how well the business is streamlined. It tries to look for patterns in the historical data and business strategies to predict future outcomes. Mostly performed by a data scientist in collaboration with business experts. At this level decisions could be automated using algorithms and AI tools.
Prescriptive analytics is built on top of the descriptive, diagnostic and predictive analytics by taking into consideration what has happened, why it has happened along with possible what if scenarios. And prescribe actions for human decision to venture into future regarding what should be done. These could be optimisation challenges or financial reformations. Its very difficultly to build prescriptive models. They are very complex and should take into account predictions, the environment, business rules, algorithms and computational simulations.
Current state of AI is is built from years of understanding the statistical learning principles embedded in the modern computational methods and gains in chip technology to perform a large number of these complex calculations resulting in solutions in quick time that augment human intelligence and perform decisive tasks towards achieving the goal. AI includes both Machine Learning(ML) and Deep learning(DL). ML is part of AI and includes Deep learning. ML is more akin to statistical learning where as deep learning tries to emulate the neural architecture in having multilayered perceptron (similar to neurons in the brain) which produce outputs to a given set of inputs.
AI can solve various kinds of problems and they are classified into different types based on the type of algorithm they use. The problems tackled by AI can be broadly classified as supervised learning, unsupervised learning and reinforcement learning. And some times can blend supervised and unsupervised methods to create semi-supervised learning. Here briefly described are the different types of machine learning methods.
In supervised learning you need to provide the inputs variables in the training data set so that the the the algorithm can train on predictions outcomes supervised by the given dataset. Supervised learning, consist of methods like regression and classification. A prerequisite for supervised learning is that you know your labels and understand the possible outcomes. Given an input and output variables the systems learns to map the correct output variables to a given input in an iterative way till the algorithm makes a prediction unit it reaches acceptable accuracy.
Unsupervised learning is a method where you give only input data without the output labels in your training dataset and you predict the possible outcomes. This includes lot of algorithms for clustering and aggregating data into groups and the hierarchical relation between the clusters or the related data points. This is a way to extract how a group of customers work or how a specific customer segment contributes to the revenue growth.
Reinforcement learning the part of artificial neural networks and how they are used to perform a specific behaviour by coding action and reward states in a given environment that unites functional approximations for the right predicted actions. Each successful step reinforces the system to evolve to the possible solutions. Famously used in developing Deep mind’s game ‘Alpha Go’, which beat world champion. Reinforcement learning can very well be applied in complex problems where immediate actions produce delayed returns. For example in real world scenarios in simulating what effects will the optimisation process in a company can have in building new plants, effect on supply chain in the long run and subsequently the capital returns.
Steinentorstrasse 35 , 4051 Basel, Switzerland