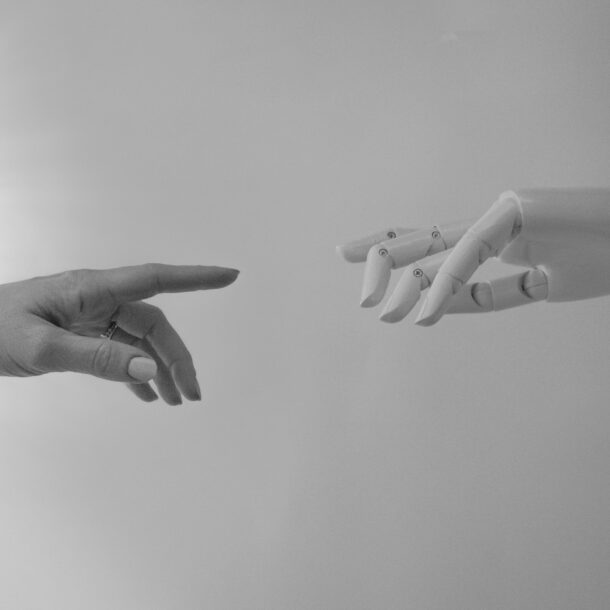
- +41 41 201 88 44
- [email protected]
- Mon-Fri 8am - 6pm
New hurdles are created by urbanization’s continuing rise. 55% of people on earth live in urban areas, according to the Department of Economic and Social Affairs of the United Nations. By 2050, this is anticipated to increase to 68%.
The big cities’ expanding populations and peripheral cities’ quick growth will cause a sudden surge. As a result, there will be pressure for: (1) environmentally friendly projects that call for more and better infrastructure in the constrained space; and (2) higher quality of life for city people at a lower price.
A “Smart City” is an urban region that uses information and communication technology to support governing systems, foster economic growth, and enhance the quality of life. For instance, a local authority might connect its energy and transportation grid systems, construct buildings with sensors for energy efficiency, and create communications to improve the monitoring and accessibility of healthcare, emergency, and other public services.
Smart City technologies need to analyse huge amounts of data, or “Big Data,” to work. The three “Vs” have been used to define big data as “high-volume, high-velocity and/or high-variety information assets,” which refers to enormous datasets that are processed fast (using algorithms) and that utilise many data sources, including mixing diverse datasets.
Artificial intelligence (AI) and big data are related. When non-human technology is used to “learn from experience and emulate human intelligent behaviour,” this is referred to as artificial intelligence (AI). Large amounts of Big Data can be effectively sorted by using AI to produce data forecasts and affordable solutions that power Smart City technology.
Applications that harmonise the experience of its users might be useful in cities with extensive transit infrastructure and systems. Via their smartphone applications, passengers of trains, buses, and autos may share real-time information about delays, breakdowns, and less crowded routes. Encouraging other commuters to change their preferred travel routes, might relieve traffic in the future. Cities may change public transportation routes and schedules and assign more precise infrastructure budgets by gathering and analysing data on how people use the system.
The same sensor and camera networks may be utilised to prevent crime and save lives. Emergency services may use traffic signals and congestion data to travel more quickly and safely to their destinations. To create future predictive and preventative actions, cities can collect data on accidents or select other characteristics to monitor.
In key building places, sensors may be installed to track energy use and forecast customer behaviour. For instance, store owners and merchants may employ sensors to measure when people are most likely to come and utilise their establishments, as well as the locations where they tend to congregate. The data produced by AI may be used to track daily, monthly, and seasonal variations and make reliable forecasts.
Power grid security and performance management might both be improved by AI and smart cities. Large amounts of data from smart metres may be read by smart grids (power networks, such as generating plants, that are incorporated with computer technology), which can then be used to analyse and forecast load clustering and demand response. On these grids, prediction models may be built up to predict the cost and demand for energy over a given period. According to research, these models are more accurate in forecasting price and load than the competition.
In the context of smart cities, AI may analyse personal data (for instance, to provide and monitor electricity usage in a person’s home, or to track movements and offer geo-targeted advertisements to potential customers passing through metropolitan areas). For safety and personalization purposes, it may also involve tracking and monitoring people as they move through public locations using face recognition technology. There are several extra issues with privacy and data governance when AI is processing personal data.
There could be more difficulties with the algorithm’s fairness and dependability. For instance, it would be hoped that the dataset used to train facial recognition technology for use in policing and public safety would have a sufficiently wide representation of various demographics, so that it correctly identified people of various racial and ethnic origins rather than one specific ethnic group more reliably.
Steinentorstrasse 35 , 4051 Basel, Switzerland